Research at the School of Information Technology
Research at the School of Information Technology (ITE) contributes to solutions of societal challenges within the University’s focus areas Health Innovation and Smart Cities and Communities.
At ITE, research is conducted within aware intelligent systems, smart electronic systems, cyber physical systems and digital service innovation. These four areas constitute the four technology areas of ITE.
Research goal
The research goal within ITE is to provide and develop knowledge (solutions, theories, methods, tools) that are relevant for the creation of innovative IT-based products and services – from enabling technologies, via system solutions and applications, to value-creating IT utilisation. With this, we intend to contribute to strengthening the competitiveness of Swedish industry.
The School’s researchers are part of the following research programmes
LeaDS – Learning in a Digitalised Society
M4HP – Movement for Health and Performance
REBEL: Re-Imagining Future Smart Living – Beyond the Living Lab
Research projects and news
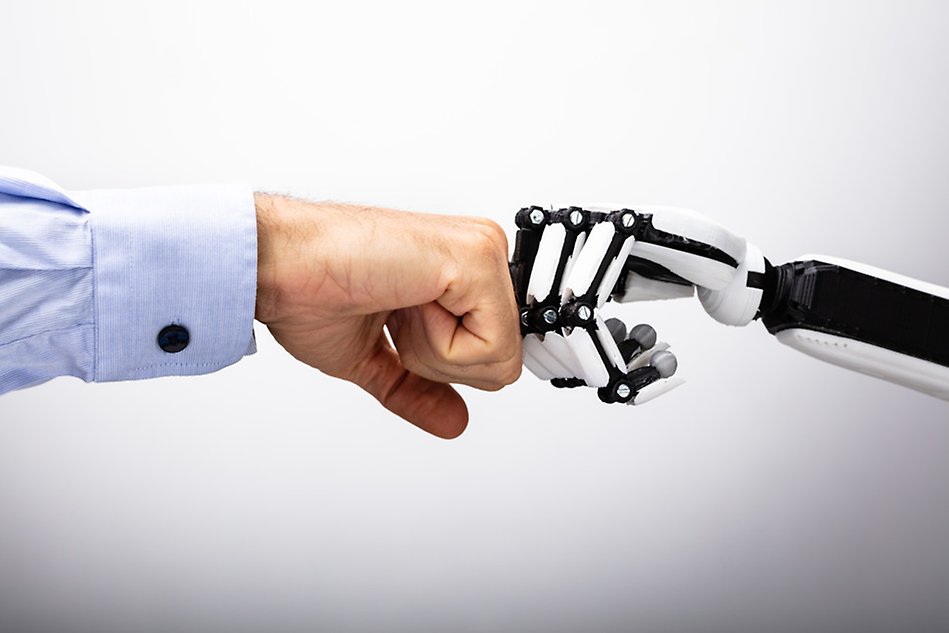
The University's popular science research magazine Samspel contains news about current research and portraits of all our professors.
Technology areas
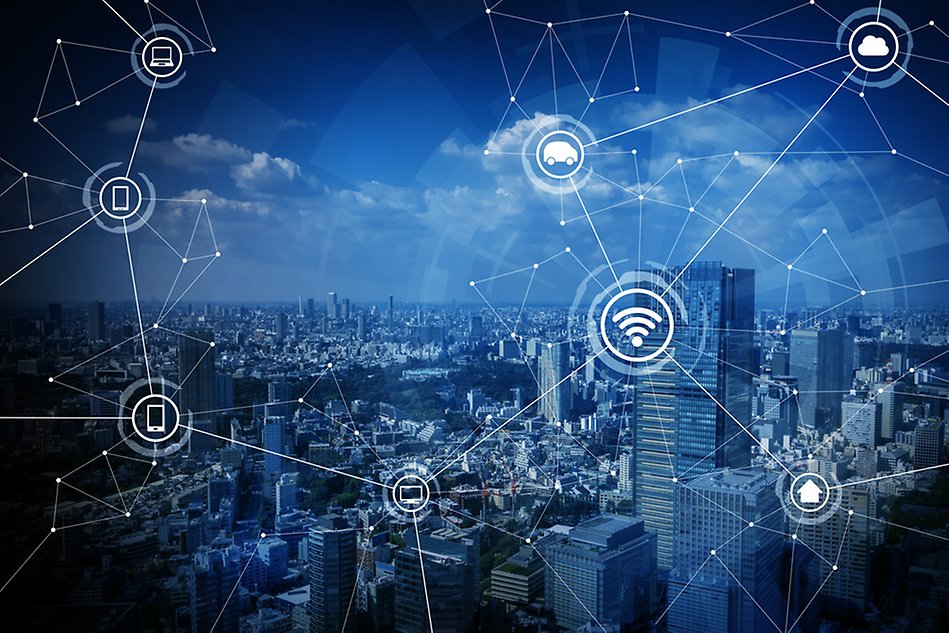
Research at ITE is conducted within four technology areas. The competence in these technology areas include signals and systems, informatics, mathematics, computer science and engineering, forensics, electronics and physics. These competences form the research at ITE.
Publications
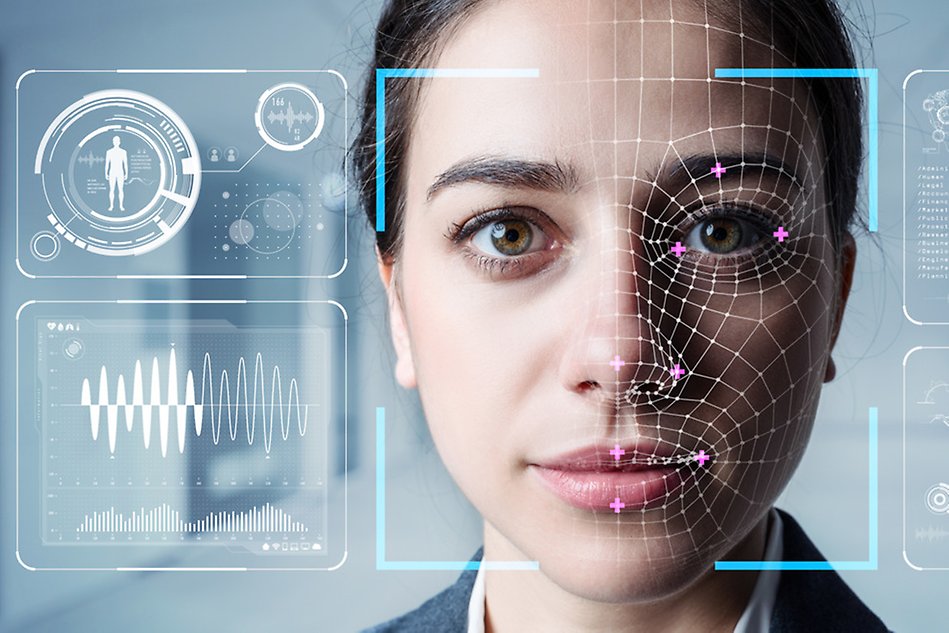
Here is a compilation of the very latest publications from ITE in DiVA and doctoral and licentiate dissertations from the School.
Research centres and profile
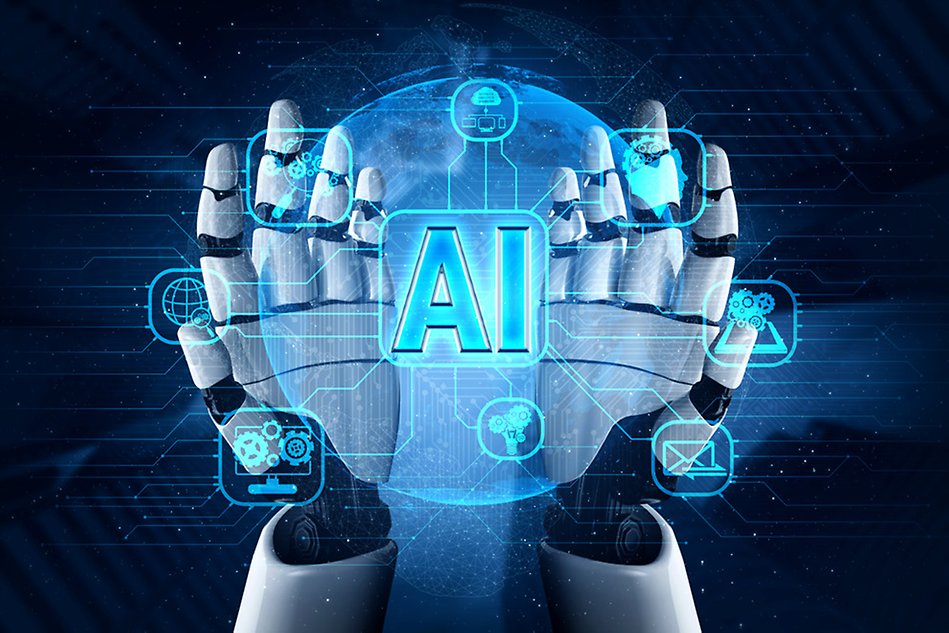
ITE gathers knowledge, research and development in a research and education centre for AI, a research profile within information driven care and in an industrial research centre that connects AI and machine learning with health data.
Doctoral education and industrial graduate school
Doctoral education in Information Technology aims to produce well-trained scientists, educators and future leaders in information technology. The industrial graduate school (EISIGS) provides a good environment for qualified and independent researchers (PhDs) to develop within embedded and intelligent systems research.
Doctoral education in Information Technology
Embedded and Intelligent Systems Industrial Graduate School (EISIGS)
Collaboration
ITE shall be characterised by well-developed research collaborations with the business sector and the public sector. Through our joint competences we can be an attractive partner and deal with projects where the whole range is treated, from enabling technologies – like low-power technologies and semiconductor sensors – to value-adding IT use, considering user aspects. In between, system and application aspects are treated, that is intelligent algorithms, application-specific computer architectures and efficient interconnection technologies.
Our strategy is to focus on a limited number of application areas in which we get recognised by industry and society as a key player and natural cooperation partner. Currently, the selected application areas are: health technology, traffic and transport systems, process industry, high-performance signal processing applications, experience industry, together with the (non-application) area of ground breaking electronics.