Improving forklift efficiency through data-driven innovation
In today’s digital world, monitoring machine productivity is more important than ever. Machine Activity Recognition (MAR) uses data to improve the efficiency of industrial equipment. In August, Kunru Chen defended her doctoral thesis on forklifts. Her research, which looks at the manufacturer’s perspective rather than the user’s, helps manufacturers understand how their machines are used worldwide.
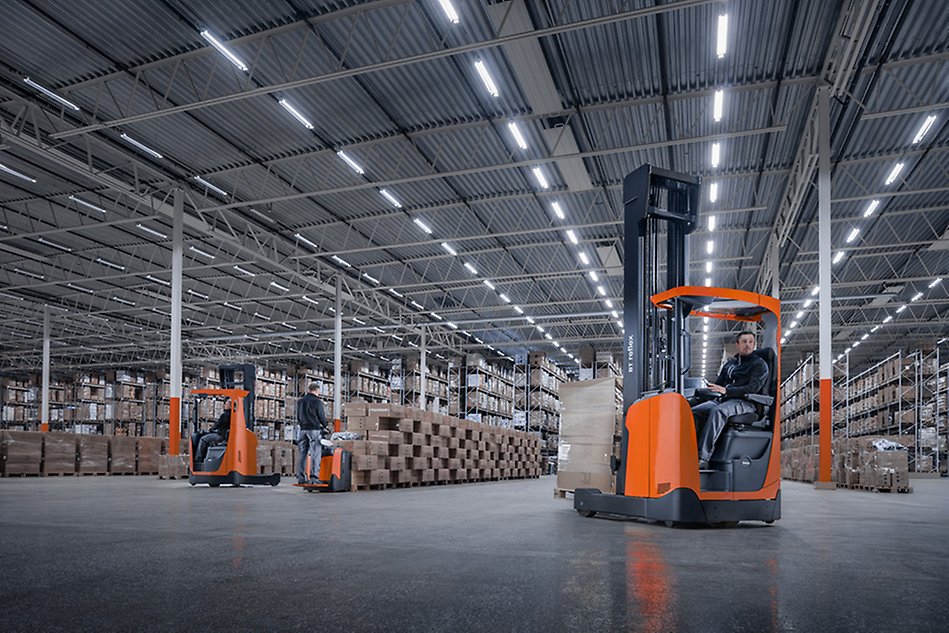
“The tests show that our method has great potential for real-world applications and can be adapted to other situations.”
Kunru Chen, Research Engineer
Forklifts are used in various ways and are influenced by factors such as the operator, the load, and the working environment. Their flexibility creates many challenges. Traditional methods, where machines are trained with predetermined data through supervised learning and sensors, often struggle to manage the variations, especially when the forklifts are used in different environments.
“In my thesis, I have developed neural network methods to recognise recurring forklift activities in real-world settings”, explains Kunru Chen.
This research is highly significant for the industry. Helping manufacturers better understand how their equipment is used can improve productivity monitoring and cost savings.
“In the long run, this could contribute to creating a more efficient and resilient use of equipment within the industry”, says Kunru Chen.
Innovative methods for activity recognition
In her research, Kunru Chen introduces three methods for analysing data collected directly from forklifts.
The first method uses autoencoders, which are algorithms designed to detect patterns in data. Initially, they are trained with unlabelled data that has no predetermined categories. The system is then refined using pseudo-labelled data with temporary categories to guide the algorithm’s further learning. This approach of generating categories often yields better results than traditional models that rely solely on pre-labelled data.
“This allows us to extract more detailed features, improving our ability to recognise various activities”, says Kunru Chen.
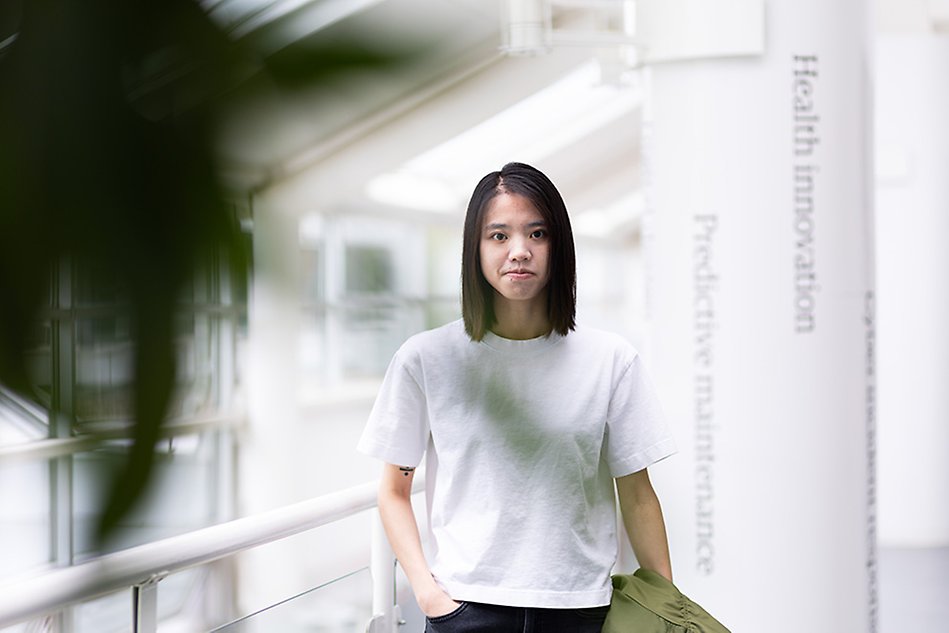
Kunru Chen began her research journey as an exchange student at Halmstad University in 2015.
The second method uses Gated Recurrent Units (GRUs), which are networks designed to recognise activities within different contextual settings. This allows the model to learn various activity categories and their corresponding transitions, resulting in stable activity recognition outcomes.
The third method, known as domain adversarial training, addresses the challenge of limited labelled data when adapting models to new environments. This technique allows a model trained in one context, such as a specific warehouse type, to perform effectively in various other settings, such as different types of warehouses or operational conditions.
“This technique creates representations that work well across different environments, allowing us to generalise knowledge about understanding equipment usage even with limited labelled data”, Kunru Chen explains.
The results, tested with industry partners on actual forklifts, have been promising.
“The tests show that our method has great potential for real-world applications and can be adapted to other situations”, says Kunru Chen.
Collaboration and future research
In her current project, Kunru Chen has collaborated with Toyota Material Handling Sweden AB, and this partnership has been crucial to the project’s success. There are also exciting opportunities for further development.
“We have recently received approval for two new applications, so our team will continue to explore this field, deepen our insights, and build more industry collaborations”, says Kunru Chen.
A rewarding journey
Reflecting on her academic journey, Kunru Chen describes applied research as both more rewarding and more challenging than she had anticipated. She began her research journey as an exchange student in 2015 when she came to Halmstad University to pursue a Master’s Degree in Information Technology. During this time, Kunru Chen developed a strong interest in industrial data applications, which has led to her current doctoral work.
“When my supervisors offered me the opportunity to join a larger project as part of my doctoral studies, I immediately accepted! It has truly been a rewarding journey”, concludes Kunru Chen.
Text: Anna-Frida Agardson
Top picture: Toyota Material Handling Sweden AB
Portrait photo: Anna-Frida Agardson