Joint human-machine learning can improve district heating
By combining machine learning and human knowledge in self-monitoring and intelligent systems, failures and deviations can automatically be detected. Research at Halmstad University shows that when these systems are applied to district heating, money can be saved and the efficiency can be increased – leading to a more sustainable energy supply.
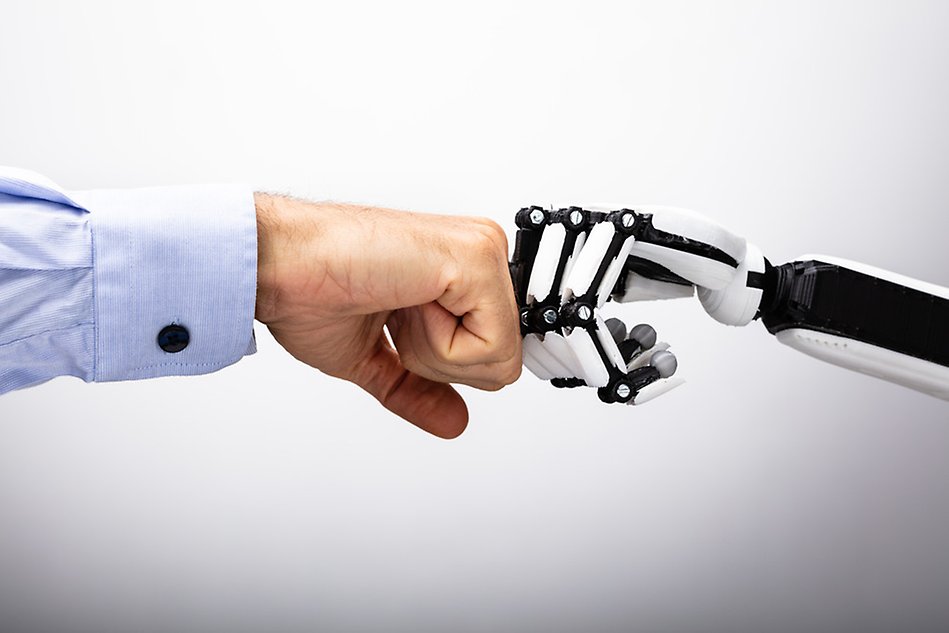
Many industries today produce and save large amounts of data from their operations. By using data driven models, the operations can continuously be monitored and adjusted for maximum efficiency. Ece Calikus is a PhD student working with research and development of so called self-monitoring systems that uses artificial intelligence, more specifically machine learning, in combination with human knowledge for industrial applications.
“The ability to diagnose deviations and predict faults effectively is an important task in various industrial domains for minimising costs and productivity loss and also conserving environmental resources”, says Ece Calikus, PhD student at Halmstad University.
An intelligent and self-monitoring system is when industrial equipment monitors its own operation. It can learn over time and automatically identify problems or deviations. There is however always a need for human interpretation and evaluation of these systems. Human experts can add other aspects, for example business and societal aspects, to achieve a holistic improvement of the industry's operations.
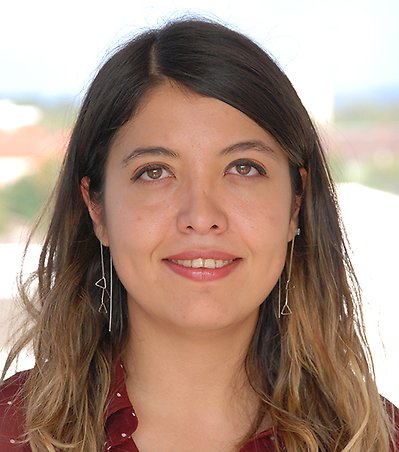
“The goal with joint human-machine learning is to create a collaborative process where machines and humans learn from each other", says Ece Calikus.
About Ece Calikus
When and how did your interested in ML/AI/intelligent systems begin?
I have been interested in AI since my childhood. My childhood interest was of course based on books and movies, more science fiction rather than scientific. I started to get involved in these fields when I took related courses and projects during my bachelor studies. But, the real turning point was watching IBM's Watson winning the Jeopardy competition. After that, I decided to be one of the ones who create machines like Watson.
When and where are you born?
I was born in Turkey on March 6, 1990.
What is your educational background?
I received my B.Sc. degree in computer engineering from Istanbul Technical University, Turkey, in 2013, and my M.Sc. degree in computer science from the University of Greenwich, UK, in 2015.
When did you come to Halmstad University?
I came here in September 2016.
How come you chose Halmstad University for your doctoral education?
I had been looking for a PhD position, in which the research topic is related to machine learning and AI. I saw the vacancy for my current position in a google group (ML news) posted by my current supervisor. By the time I saw the post, I had already accepted another PhD position at the University of New Castle, UK. However, I liked the research topic of my current project much more. So, I decided to choose Halmstad University instead.
What are your future plans?
I will continue my research on self-monitoring and joint human-machine learning and extend my licentiate work to a PhD thesis with new contributions. My plan is to defend my thesis at the end of 2021. After my PhD, my plan is to continue my career in academia. I'd like to extend my research direction to cover applications that have social impacts, and also to tackle the question of "how to make ML systems fairer and more equitable for society”.
Humans and machines work better together
The combination of machine learning models and human expertise is called joint human-machine learning.
“The goal with joint human-machine learning is to create a collaborative process where machines and humans learn from each other. People should be able to observe, interpret and learn from the self-monitoring results, as well as provide domain knowledge or feedback to the intelligent system”, says Ece Calikus who published her licentiate thesis on the subject in 2020.
Ece Calikus is a PhD student at the School of Information Technology at Halmstad University. She is working at the Center for Applied Intelligent Systems Research (CAISR) in a research project called Self-Monitoring for Innovation (SeMI). Her licentiate thesis focuses on generating a real-world self-monitoring system that can learn data and their relations over time and detect anomalies and discover faults using joint-human machine learning.
“Throughout the thesis, I have described a number of different approaches, each designed for the needs of a self-monitoring system, and I have composed these methods into a coherent framework”, says Ece Calikus.
About the Center for Applied Intelligent Systems Research (CAISR)
Ece Calikus works at the Center for Applied Intelligent Systems Research (CAISR) at Halmstad University. Here, researchers work together with partners from the private and public sector in joint projects to develop industry and society. One of the projects at CAISR that Ece Calikus is working with is SeMI (Self-Monitoring for Innovation) which is funded by the Knowledge Foundation. The goal with SeMI is to develop self-monitoring systems for different industries, while transferring knowledge and experience gathered from one industrial domain to other domains.
Improving district heating by automatic detection of faults
Self-monitoring systems can be applied to a range of different industries. Ece Calikus is focusing her research on applying these intelligent systems to district heating in order to increase the efficiency in that domain. The research is done in collaboration with two district heating companies, HEM and Öresundskraft. They have provided both data used in the research and knowledge about district heating.
“When applied to district heating, the self-monitoring system can automatically detect faults and abnormal patterns in district heating networks by comparing heat demands among different groups of customers”, says Ece Calikus.
When faults and deviations are detected in time, the districts heating companies can use this information for further investigation, to repair faults and optimise their operations.
“Future energy systems are facing critical challenges such as the steady growth of energy demand, energy resource depletion, and increasing emissions of carbon dioxide and other greenhouse gases. District heating can play a vital role in the implementation of future sustainable energy systems that will contribute to a decrease in carbon emissions. However, the current generation district heating technologies have problems that prevent them to operate efficiently. I hope that my research can lead to more efficient district heating and thus contribute to a more sustainable energy supply system”, says Ece Calikus.
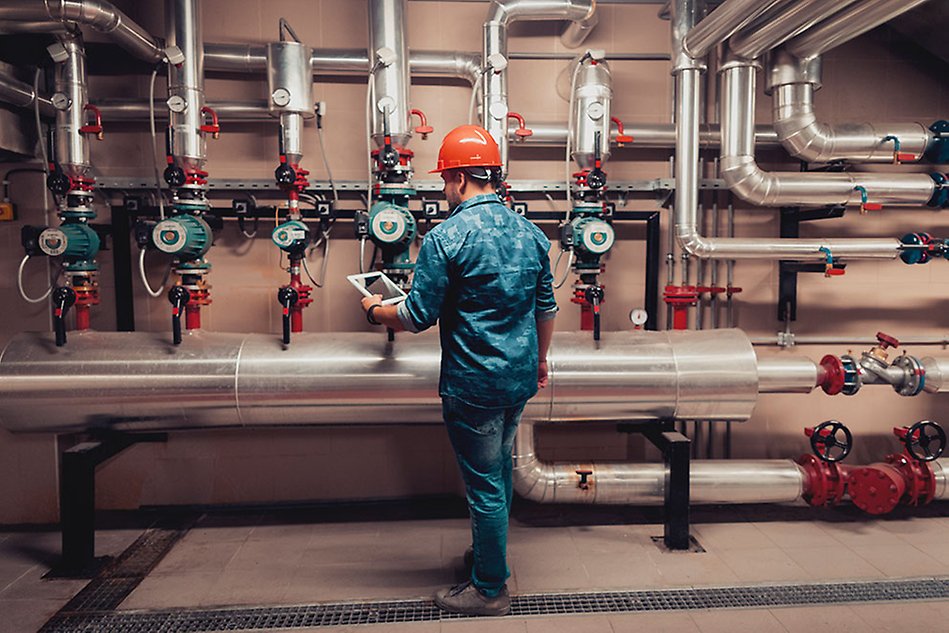
When faults and deviations are detected in time, the districts heating companies can use this information for further investigation, to repair faults and optimise their operations.
Her method takes minutes – instead of years
One goal with Ece Calikus’ research has been to automate a very time-consuming manual method of analysing heat load patterns for identifying unsuitable behaviours of buildings in a district heating network.
“The manual analysis was restricted to 140 buildings and took several years for a group of researchers to conclude. My goal was to automatise their manual effort with a data-driven approach so that it can be applied to thousands of buildings in less than 10 minutes”, says Ece Calikus.
She expected to discover the same patterns they did, but automatically and much faster. However, Ece Calikus and her colleagues’ method not only successfully captured the same heat load patterns, but also discovered novel and abnormal patterns that have not been known before.
“It was surprising and very exciting to see that our results provided completely new knowledge to the district heating community. Furthermore, my work also revealed the limitations of the previous knowledge within this area and the importance of intelligent systems for energy efficiency”, says Ece Calikus.
Text: Louise Wandel
Photos: Roland Thörner and iStock
About the licentiate thesis
Thesis title: Self-Monitoring using Joint Human-Machine Learning: Algorithms and Applications External link.
- Presentation: February 25, 2020, at Halmstad University
- Opponent: Dr. Rita Ribeiro, University of Porto
- Supervisors at Halmstad University:
- Sławomir Nowaczyk, Professor
- Onur Dikmen, Senior Lecturer
- Stefan Byttner, Senior Lecturer
The research was funded by the Swedish Knowledge Foundation.