Research keeps AI compatible with smart devices
The smart devices we use in our daily lives keep getting smarter and are demanding more and more out of the hardware. How can we make sure that these devices are compatible with the artificial intelligence needed to keep them functioning, without having to increase the hardware capacity? This is what Nesma Rezk, PhD in Computer Science and Engineering, has been researching in her dissertation.
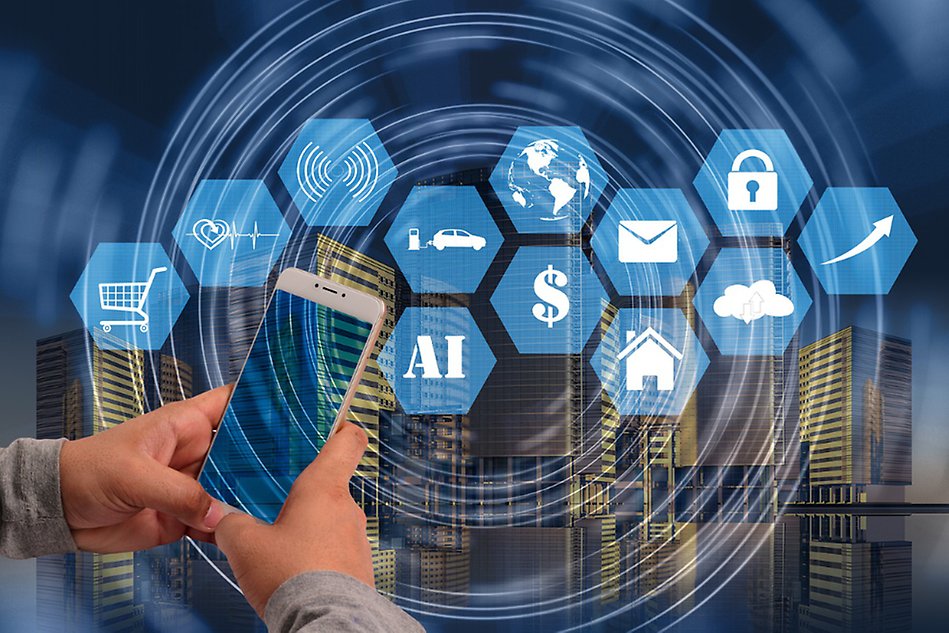
“By realising deep learning applications on embedded platforms and making this realisation efficient, fast, and robust, we can integrate AI into every aspect of our daily lives.”
Nesma Rezk, PhD in Computer Science and Engineering
Nesma Rezk’s thesis is about implementing deep learning applications on embedded platforms, which is any type of computer system with a dedicated function, such as a smart watch or an autonomous car. Deep learning is a type of artificial intelligence (AI) technique that teaches computers to learn by example. This technique is for example what makes it possible for a driverless car to recognise different traffic signs, and the technology can be found in everything from smart home gadgets to healthcare instruments.
“The technique raises questions about how you can use these implementations, ensure their efficiency, and cope with the technological advances in deep learning algorithms that are continuously being developed to be more intelligent”, says Nesma Rezk.
About Nesma Rezk
Nesma Rezk was born in Cairo, Egypt. She received both her Bachelor and Master of Science degrees from the Faculty of Engineering at Ain Shams University in 2010 and 2015, respectively.
In 2016, she came to Halmstad University for her doctoral studies. During her initial time here, Nesma Rezk collaborated with researchers from Amrita University in India, and later with a research group at KTH Royal Institute of Technology.
Nesma Rezk has a PhD in Computer Science and Engineering. In the future, she hopes to keep working within academia, mainly with teaching.
Enables AI in daily life
Even though there are advanced hardware platforms offering powerful performance, the high demands of deep learning models for the hardware’s computational and memory resources still pose a challenge – and if the hardware and models are not sufficiently compatible, this can limit the efficiency of the resulting implementations.
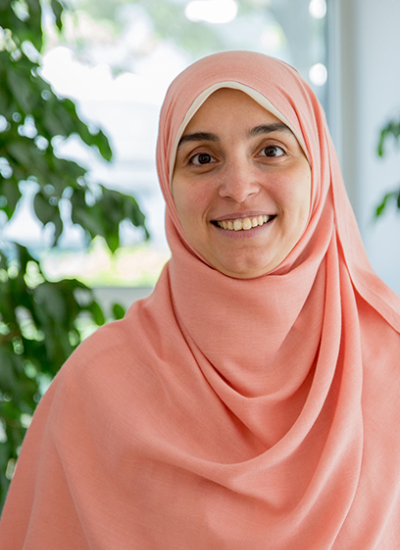
Nesma Rezk.
Nesma Rezk was surprised to see that in many cases, it was possible to compress the deep learning model to less than a quarter of its original size, and still have the model functioning correctly.
“Deep learning applications should not be deployed directly to embedded systems. A prior step called algorithmic optimisation should be applied first. Algorithmic optimisations are methods that decrease the requirements of deep learning applications to make it possible to run them on resource-limited platforms while keeping them functioning correctly”, she explains.
Nesma Rezk believes that her research can be part of the link between deep learning and our daily life.
“By realising deep learning applications on embedded platforms and making this realisation efficient, fast, and robust, we can integrate AI into every aspect of our daily lives”, she says.
Text: Emma Swahn
Photo: Helena Bengtsson, Pixabay
About the PhD defence
The public defence took place on December 15, 2022, at Halmstad University.
The dissertation’s title: “Deep Learning on the Edge: A Flexible Multi-Level Optimization Approach” External link, opens in new window.
- Opponent: Antonio Carlos Schneider Beck, Professor, The Federal University of Rio Grande do Sul (UFRGS), Rio Grande do Sul, Brazil
- Supervisors: Magnus Jonsson, Professor, and Mahdi Fazeli, Senior Lecturer, both from Halmstad University