AI technology for a fossil-free future
In the field of transportation innovation, Mohammed Ghaith Altarabichi’s,who recently defended his doctoral thesis in machine learning at Halmstad University, research plays a key role towards a greener, more sustainable future. As a part of the EVE project, a collaboration between Halmstad University and the Volvo Group, he focuses on enhancing the lifespan of electric buses using AI and predictive maintenance. By predicting issues and maintenance needs before damage occurs, he contributes to improving the reliability and efficiency of these vehicles.
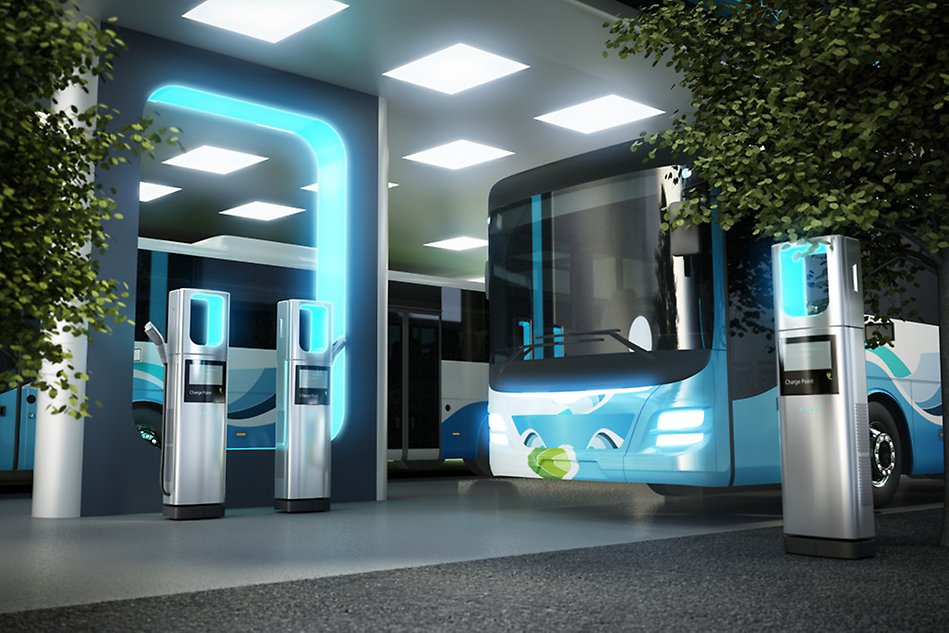
“By optimising battery performance and generalising machine learning models to previously unexplored domains, we’re taking meaningful steps towards reducing environmental impact and encouraging wider adoption of electric vehicles.”
Mohammed Ghaith Altarabichi
In the EVE project the researchers, including Ghaith Altarabichi, focus on developing efficient maintenance strategies for heavy vehicles like buses. They utilise AI technology on critical components of the vehicle’s electrical systems to enhance their efficiency.
“The research contributes to promoting electric mobility services, addressing sustainability challenges within the transport sector, and facilitating the transition to fossil-free roads. All of this falls within the broader scope of the EVE project,” says Mohammed Ghaith Altarabichi.
Improving predictive maintenance with advanced AI models
Delving into Evolutionary Deep Learning (EDL) and Evolutionary Machine Learning (EML), Mohammed Ghaith Altarabichi endeavours to develop AI models capable of accurately predicting maintenance needs. By applying Evolutionary Computation (EC) techniques, he aims to create models that precisely forecast maintenance requirements, thereby preventing breakdowns and ensuring smooth machinery operation.
“One of my main goals has been to make EDL and EML algorithms more practical by reducing computational costs and utilising Evolutionary Computation methods”, Mohammed Ghaith Altarabichi says and continues: “To do this, I’ve introduced approximate models to make certain parts of Deep Neural Network (DNN) design better in ways that haven’t been tried before.”
Deep Neural Networks (DNN), Evolutionary Deep Learning (EDL) and Evolutionary Machine Learning (EML)
Deep Neural Networks (DNNs) are artificial intelligence (AI) that mimics how the human brain works to solve problems. DNNs have layers of artificial neurons that work together to understand and analyse data.
Methods such as EDL and EML optimise the computer’s ability to learn from past data, improving its capacity to anticipate maintenance requirements. These approaches involve using EC techniques to tailor AI models for predictive maintenance. By studying these techniques, Mohammed Ghaith Altarabichi hopes to create AI models that accurately forecast maintenance needs, thereby preventing breakdowns and ensuring machinery operates smoothly.
Uncovering patterns crucial for development
Mohammed Ghaith Altarabichi’s research addresses computational challenges inherent in EDL and EML algorithms, significantly improving their efficiency while maintaining high-quality results. By identifying invariant features and patterns applicable to various settings for hybrid buses, his work can potentially optimise electromobility systems.
“Additionally, my contributions extend into the field of deep neural network design, where I’ve introduced a range of evolutionary computation algorithms,” Mohammed Ghaith Altarabichi says and continues: “By identifying invariant features, I’ve uncovered patterns that generalise well to new settings for hybrid buses, including different configurations and operating conditions. This finding is crucial for developing more reliable and efficient electromobility systems.”
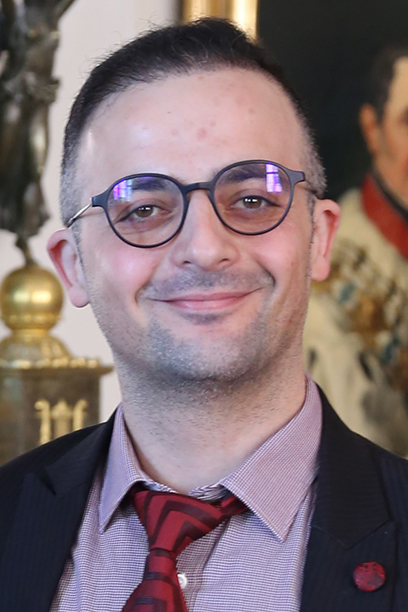
On February 16, Mohammed Ghaith Altarabichi defended his thesis “Evolving Intelligence: Overcoming Challenges for Evolutionary Deep Learning”.
Adding randomness makes computers learn faster
By studying how randomness affects computer algorithms, Mohammed Ghaith Altarabichi’s research demonstrates that adding controlled randomness into deep learning systems can enhance efficiency and effectiveness.
“My research explores how randomness affects computer algorithms. I found that by using approximate fitness models, that is, simplified models that include a bit of randomness, I could make our EC algorithms work much faster and explore better ways to solve problems,” Mohammed Ghaith Altarabichi says and continues: “This unexpected efficiency gain shows that carefully adding randomness to the process of teaching computers can be helpful. My research systematically categorises and explores the significance of randomness in deep neural network design, which is used to recognise images or predict outcomes. Here, I found that adding noise to the loss function had a regularising effect on the systems that could make them work better.”
Optimising battery capacity reduces environmental impact
Through their research, Mohammed Ghaith Altarabichi and his colleagues in the EVE project hope to tackle challenges within electric vehicles (EVs), focusing on electric and hybrid buses. They have developed an algorithm that seamlessly transfers machine learning models to new situations by identifying patterns consistent across different settings. This innovation optimises battery capacity utilisation and extends their lifespan.
“By optimising battery performance and generalising machine learning models to previously unexplored domains, we’re taking meaningful steps towards reducing environmental impact and encouraging wider adoption of electric vehicles,” says Mohammed Ghaith Altarabichi.
Moreover, the research is described as the product of fruitful collaborations.
“Working closely with Volvo Buses has provided me with invaluable insights. Additionally, establishing a partnership with the University of Manchester through Professor Julia Handl, and visiting the UK during my education have been extremely enriching experiences,” says Mohammed Ghaith Altarabichi.
Text: Anna-Frida Agardson
Picture: iStock
Portrait picture: Private
About Mohammed Ghaith Altarabichi
What is your educational background?
“I have a Master’s in Computer Science from Kristianstad University in Sweden.”
When did you come to Halmstad University?
“I came to the University in 2019.”
Why did you choose Halmstad University for your doctoral education?
“The compelling prospect of collaborating with Professor Slawomir Nowaczyk significantly influenced my decision. Our initial encounter at Mälardalen University left a lasting impression, and when the opportunity arose to work under his supervision, I enthusiastically embraced it.”
When and how did your interest in machine learning and AI start?
“My fascination with employing nature-inspired algorithms to refine artificial intelligence began in 2018 upon my introduction to the application of Genetic Algorithms in solving the travelling salesman problem. This initial exposure ignited a profound interest, leading me to delve into Evolutionary Computation (EC) algorithms as a promising conduit for elevating intelligence in machine learning. This intellectual curiosity forms the cornerstone of my research pursuits, propelling my commitment to unravelling the latent potential inherent in EC algorithms within artificial intelligence.”
What are your plans for the future?
“In the future, I plan to continue my academic journey by pursuing postdoctoral research and engaging in advanced studies. My long-term goal is to play a role in shaping the future of AI-driven technologies and their applications.”
About the doctoral defence
The dissertation took place on February 16, 2024.
Thesis title:
Evolving intelligence: Overcoming challenges for Evolutionary Deep Learning External link.
Chair of the defence:
Professor Stefan Byttner, Högskolan i Halmstad
Opponent:
Professor, Niklas Lavesson, Blekinge Tekniska Högskola
Examination board:
Professor, Bing Xue, Victoria University of Wellington
Professor, John McCall, Robert Gordon University
Professor, Richard Allmendinger, The University of Manchester
Supervisor:
Professor, Slawomir Nowaczyk, Högskolan i Halmstad
Co-supervisor:
Sepideh Pashami, Associate Professor and Peyman Mashhadi, Senior Lecturer
More information
Research at the School of Information Technology (ITE)
Mohammed Ghaith Altarabichi’s doctoral thesis: Evolving intelligence: Overcoming challenges for Evolutionary Deep Learning External link, opens in new window.
På svenska
AI-teknik för en fossilfri framtid External link, opens in new window.